Navigating the Shift in Data Management
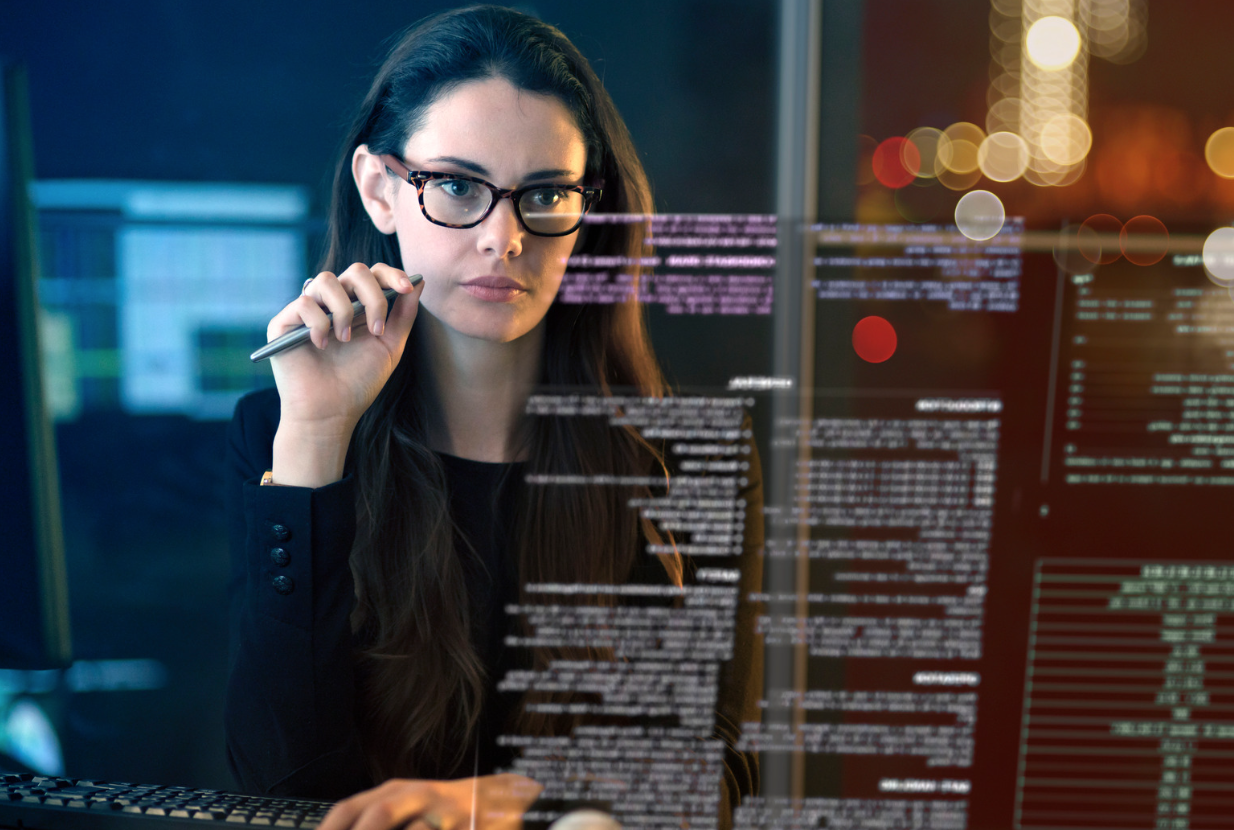
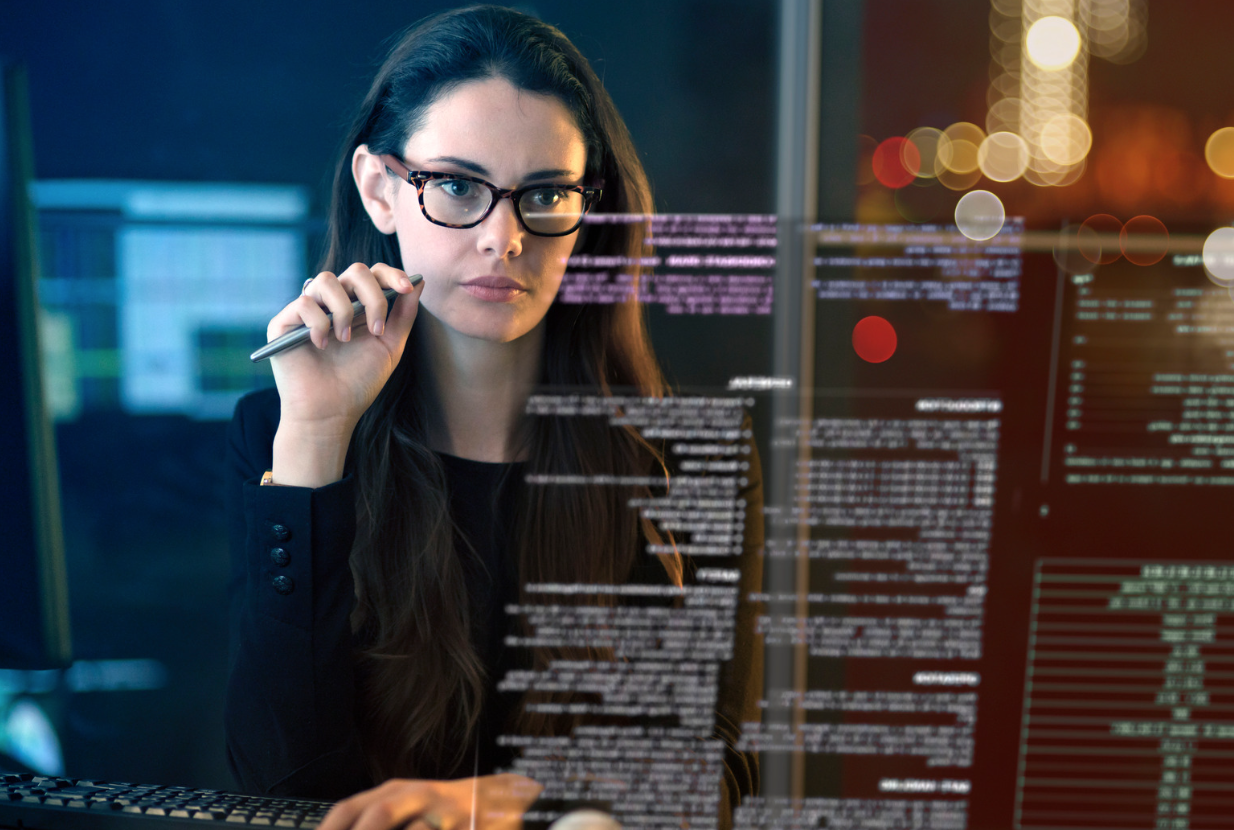
Navigating the Shift in Data Management
From Data Links to AI Insights: Are you navigating the shift in your data management for optimal business operations?
Is your data serving your business? In the early years of the 21st century, enterprises commonly used data marts and large data warehouses for storing their data. These solutions were reliable and easily accessible, but they lacked flexibility and scalability, leading to data becoming isolated. Data warehouses employed a method where data was modified to fit pre-established schemas before being stored, a process that became cumbersome as data volumes grew and schemas needed frequent updates.
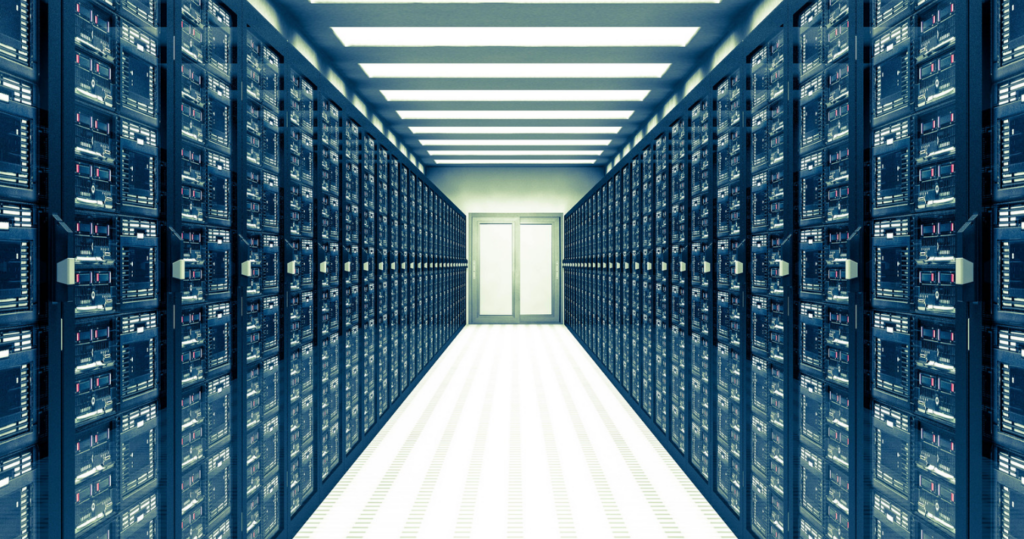
By 2010, the concept of a data lake gained traction. This method involves storing data in its raw form first and then transforming it as needed. This approach is faster since schemas don’t need constant revisions with each new data type. However, this method’s downside is the lack of clarity and quality control over the stored data.
Previously, the cost and space constraints of data storage ensured efficient data management practices, like data normalization and effective schema design. However, the advent of inexpensive and abundant cloud storage led to a shift towards the indiscriminate storage practices seen in data lakes.
Now, companies are attempting to harness the potential of this accumulated data using AI and BI tools. They are discovering that these tools struggle to extract meaningful insights from the unstructured, often irrelevant data in their data lakes, which in some cases have deteriorated into data swamps. These challenges arise because the tools are designed for and trained on clean, structured, and relevant data, unlike the chaotic mix found in real-world data lakes.
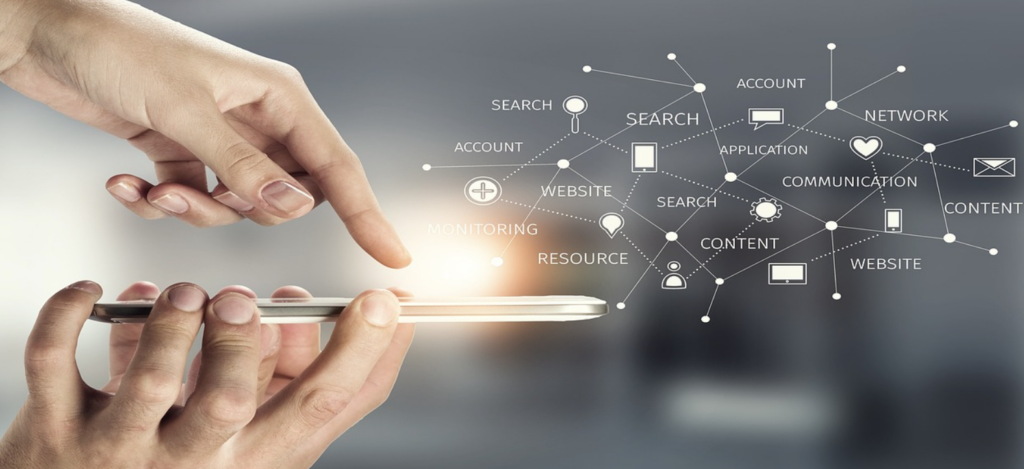
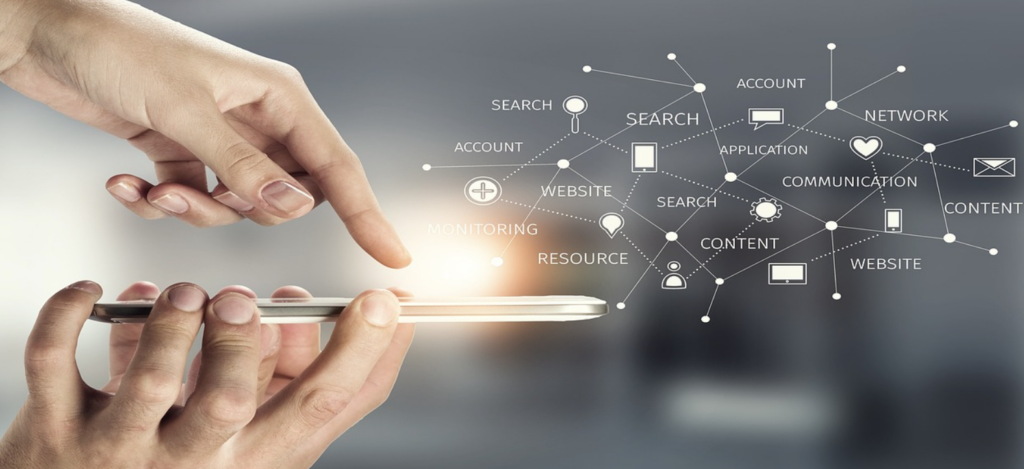
Interestingly, data lakes often lack the more nuanced, unstructured data types like sensor readings, images, or chat logs, which could be critical for specific business inquiries. The anticipated ‘magic’ of AI and BI tools often lies in these overlooked data types. When these tools can’t access relevant data, their effectiveness diminishes, leading to disappointing results.
The key to leveraging AI and BI tools effectively is to focus on quality over quantity. The data fed into these tools should be specifically relevant to the intended business objective. This approach may not lead to a smaller dataset, but relevance is crucial for the tools to function optimally. Companies often neglect their unstructured data, assuming it’s inaccessible, and vendors may not highlight this issue unless prompted by the client.
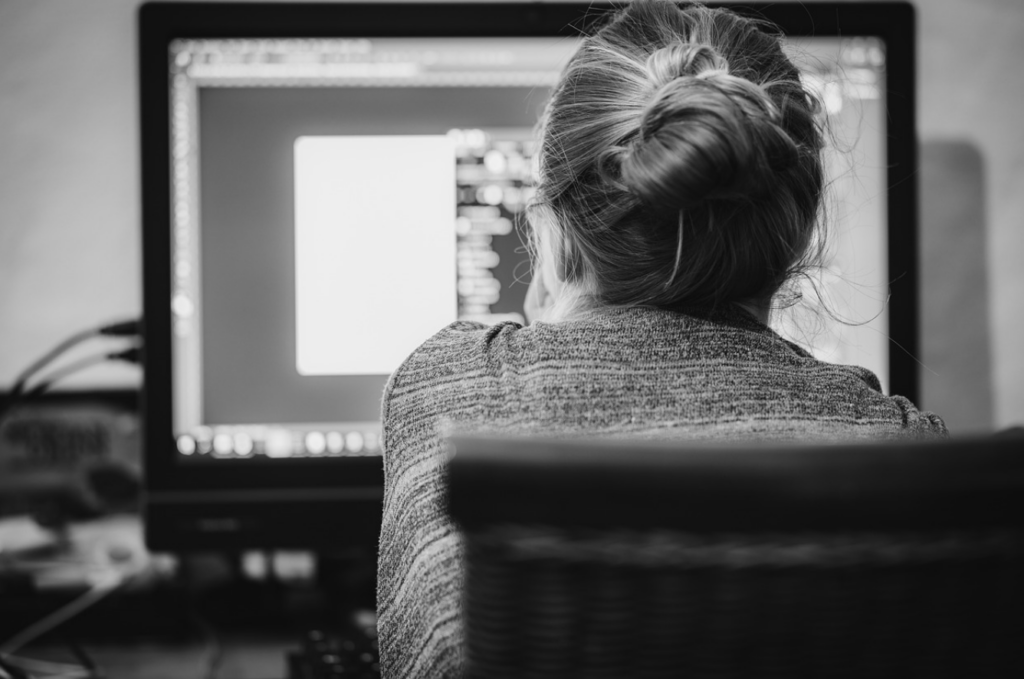
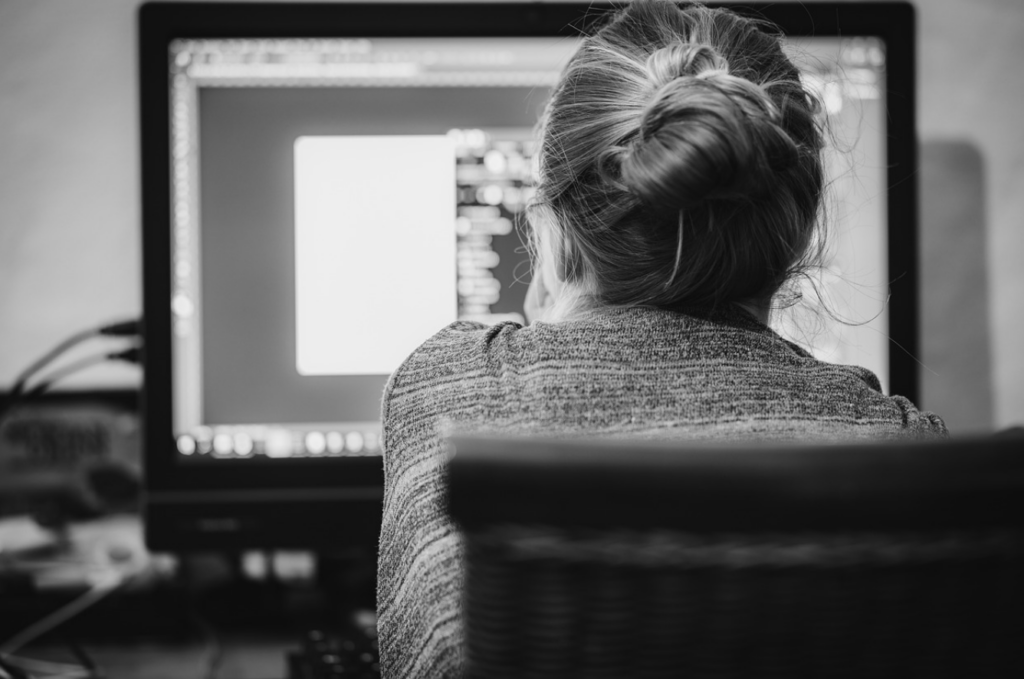
For successful AI or BI implementations, it’s essential to have a clear objective for the tool, including the expected business value, like revenue growth or operational efficiency. Identifying the exact data necessary for this goal is crucial; superfluous data can hinder performance. Companies should also explore their unstructured data, seeking assistance from data services providers if needed, to ensure all valuable data is utilized.
The era of thinking about data in terms of vast data lakes and warehouses is changing. For AI platforms to deliver their full potential, they must be carefully fed with the most relevant data, piece by piece. This thoughtful approach to data management promises more effective outcomes. To learn more about our data quality program and how it could impact your business in 2024, schedule a free consultation.
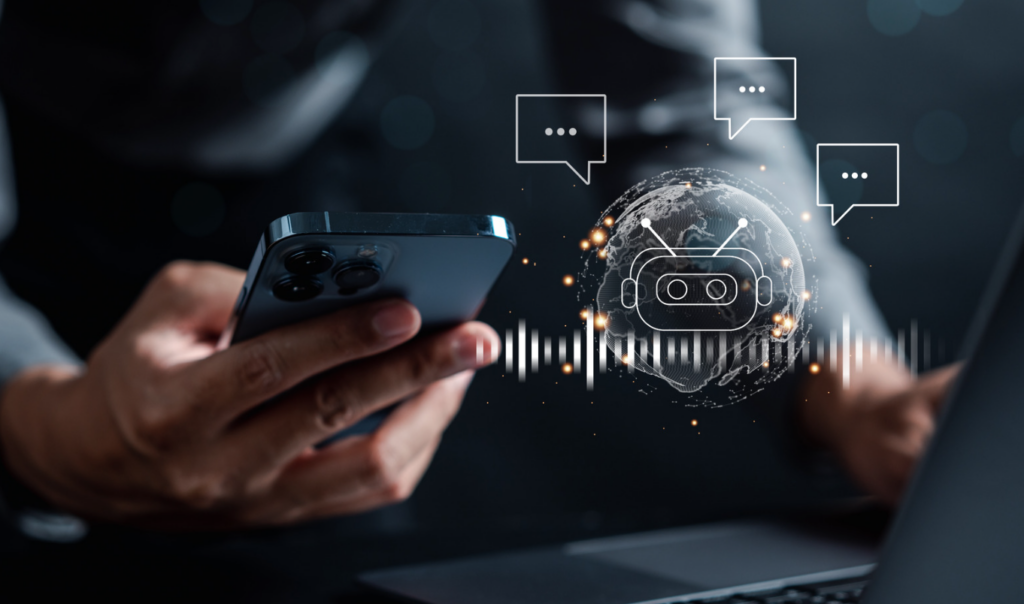
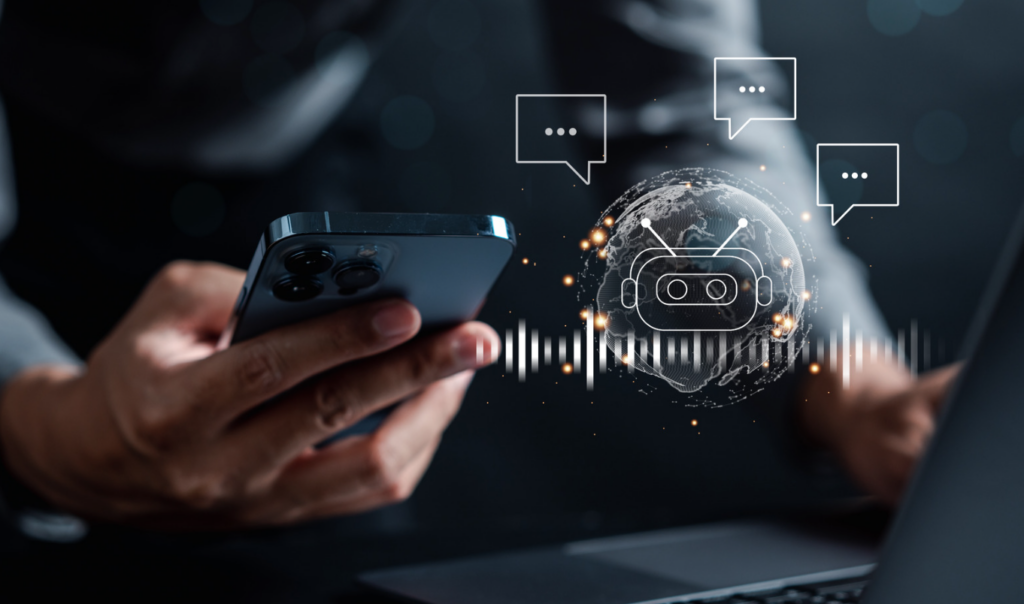
Leave a Reply